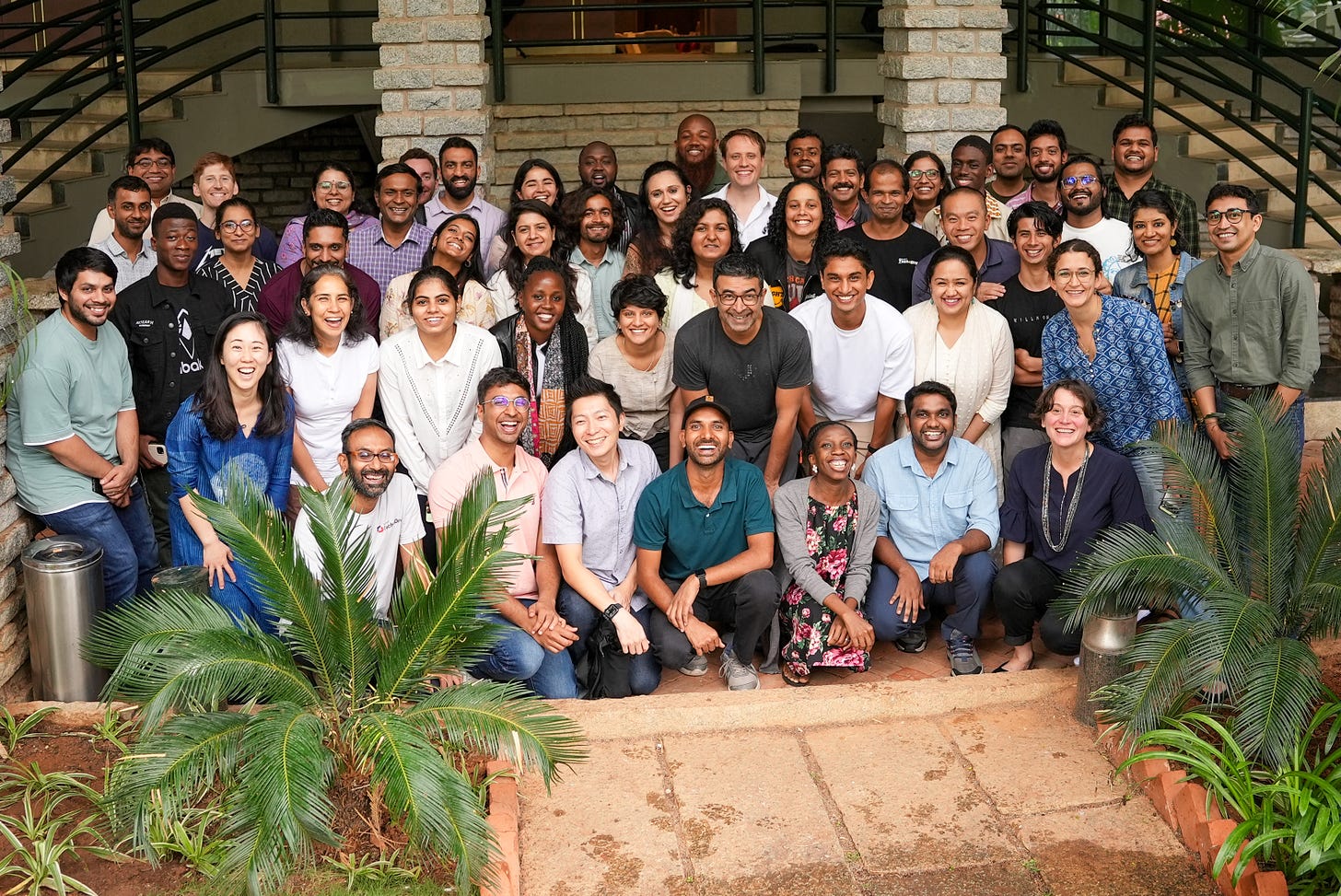
At the Agency Fund, we believe that generative AI, particularly the rise of large language models (LLMs), offers transformative potential for social sector organizations, the communities they serve, and enhancing human agency. We see human agency as the capacity of individuals to shape their own futures, make informed decisions, and access the resources they need to thrive. By helping the social sector adopt AI responsibly, our grantees and partners empower their communities, ensuring that AI-driven tools like LLMs are used not just for operational efficiency but also for enabling beneficiaries to take control of their lives.
But effectively adopting and utilizing LLMs is a process fraught with uncertainty, hurdles, costs, and technical challenges – particularly for organizations with limited resources and diverse linguistic needs.
To help social sector organizations identify and overcome these emerging pain points, we recently partnered with Project Tech4Dev to convene a two-day sprint in Bangalore, India. More than a dozen organizations gathered to share experiences, learn from each other, and address their most pressing technical problems in hands-on work sessions.
One of the sprint’s main tracks focused on using LLMs to enhance the impact and scale of chat services in the social sector.
Six organizations participated, with operations spanning Africa and India. Bandhu empowers India’s blue-collar workers and migrants by connecting them to jobs and affordable housing, helping them take control of their livelihoods and future stability. Digital Green enhances rural farmers’ agency with AI-driven insights to improve agricultural productivity and livelihoods. Jacaranda Health provides mothers in sub-Saharan Africa with essential information and support to improve maternal and newborn health outcomes. Kabakoo equips youth in Francophone Africa with digital skills, fostering self-reliance and economic independence. Noora Health teaches Indian patients and caregivers critical health skills, enhancing their ability to manage care. Udhyam provides micro-entrepreneurs’ with education, mentorship, and financial support to build sustainable businesses.
These organizations demonstrate diverse ways one can boost human agency: they help people in underserved communities take control of their lives, make more informed choices, and build better futures – and they are piloting AI interventions to scale these efforts. Each organization recently deployed their own AI-enabled chat programs that are already receiving an average of 3,000 messages per day in Hindi, Swahili, English, and a range of other languages.
Arriving in Bangalore ready to roll up their sleeves, collaborate, and learn, the participating organizations quickly identified a common set of LLM pain points – including technical issues (i.e., how their LLMs function) and higher-level challenges (i.e., how LLMs help advance their missions):
Technical issues
Understanding and adapting LLMs to individual user needs, providing relevant responses and guidance
Reducing “hallucinations,” where chatbots provide incorrect or factually wrong information
Fine-tuning responses to ensure context-specific answers
Supporting low-resource languages (for which there is limited data available to train LLMs)
Enhancing response accuracy via Retrieval Augmented Generation (RAG), a common LLM architecture
Analyzing more detailed user feedback (currently limited to thumbs up/down)
Enabling faster scaling by reducing reliance on humans to vet responses
Tackling questions outside the RAG knowledge base
Higher-level challenges
Maintaining ongoing interaction with users after initial chat
Building user trust, especially to ultimately convert users to high-trust actions like digital payments
Reaching more people and scaling operations
Retaining users and keeping them engaged, especially through features and content
Building community among users to reduce engagement gaps (especially after training ends, when engagement typically decreases)
Delivering more tailored responses that are best fit to the individual's context to boost long-term outcomes
Across these pain points, four challenges of LLM integration stand out.
Establishing baseline metrics for your LLM
All LLMs struggle with hallucination – but most organizations have only a vague sense of when, why, or how much their chatbots hallucinate. A critical first step for tracking and eventually reducing hallucinations is establishing strong baseline metrics. One popular metric for tracking hallucinations is faithfulness, which checks how accurate and reliable the generated content is based on the context provided in RAG systems. Baseline metrics enable platforms and dashboards to monitor and observe LLM outputs over time, and they can also help track costs: if left unchecked, LLM costs can grow linearly as organizations develop chatbots and build their pipelines.
Monitoring LLM performance
Another key challenge is monitoring how LLMs are performing, finding and fixing problems, and ensuring that responses are accurate and useful. At our sprint, Noora Health facilitated a workshop to highlight one such tool: Langfuse, a platform that helps monitor LLMs, address issues, and implement upgrades. As a practical case study, we brainstormed ways to integrate Langfuse into Tech4Dev’s open-source chatbot builder (Glific) and data-analysis-as-a-service platform (Dalgo), whiteboarding how the integration would work and identifying potential design partners.
Multilingual chatbots
Major LLM providers (i.e., ChatGPT) still struggle to efficiently parse and speak proficiently in less widely spoken languages, including Hindi and Swahili but also less common indigenous languages. Digital Green’s FarmerChat has achieved strong multilingualism by leveraging multiple translation services and a large curated knowledge base with many local indigenous languages represented, a challenging feat for smaller organizations. Until major LLM providers improve at multilingualism, the current best practice is a workaround: translating user queries into high-resource languages like French or English, running the translated query through your LLM, then translating the response back to the low-resource language.
Evaluating LLMs
Human evaluation of LLM performance (by taking a sample of responses and manually reviewing them) is still the gold standard. But once a chatbot is sending and receiving thousands of messages a day, organizations need automated evaluation support. A popular technique is “LLM as a judge,” or using another LLM to evaluate your LLM’s output. Organizations at the workshop demoed this technique using tools like G-Eval and RAG Assessment Scores (RAGAS) – which has a metric called “faithfulness” that evaluates how accurately an LLM’s responses match the existing knowledge base. While these tools are only half as good as human evaluation, they offer useful signals to quickly assess changes in LLM performance: a significant decrease in faithfulness, for instance, could prompt human investigation. Such methods can reduce workloads for the humans involved, but they aren’t full replacements for human judgment.
Looking ahead
These insights reflect the momentum that emerges when social sector leaders collaborate, learn, and solve problems together – and they underscore the potential for generative AI to enhance human agency. By identifying and tackling common LLM pain points, these organizations are advancing their own missions while cultivating a better digital ecosystem for the social sector and the communities they serve. Critically, they are also highlighting clear use cases for building agency-focused AI tools that empower frontline workers, improve advisory services, and bolster community support mechanisms. Rather than treating generative AI as a novel fad that can be shoehorned onto the social sector, these are practical solutions that enable people in underserved communities to take control of their lives, make informed decisions, and access the resources they need to thrive.
Even as we begin to address today’s LLM challenges, however, these technologies continue to evolve and new pain points will surely emerge. Continuous effort is critical – which is why The Agency Fund remains committed to harnessing AI for agency through our funder-doer model, staying connected to our grantees as we roll up our own sleeves, build with them, and co-learn by doing.
This means more AI sprints in the future, to help address our grantees' ongoing and future challenges so they can continue leveraging new technologies to overcome obstacles and scale their impact – so stay tuned to this space.
Building LLMs for the social sector is such an inspiring application of AI! It’s exciting to see these models helping bridge language gaps and improve decision-making in impactful ways. At Datumo, we enhance LLM-based services with our Evaluation Solution to ensure reliability and safety—learn more at https://datumo.com
Looking forward to seeing how these efforts evolve!